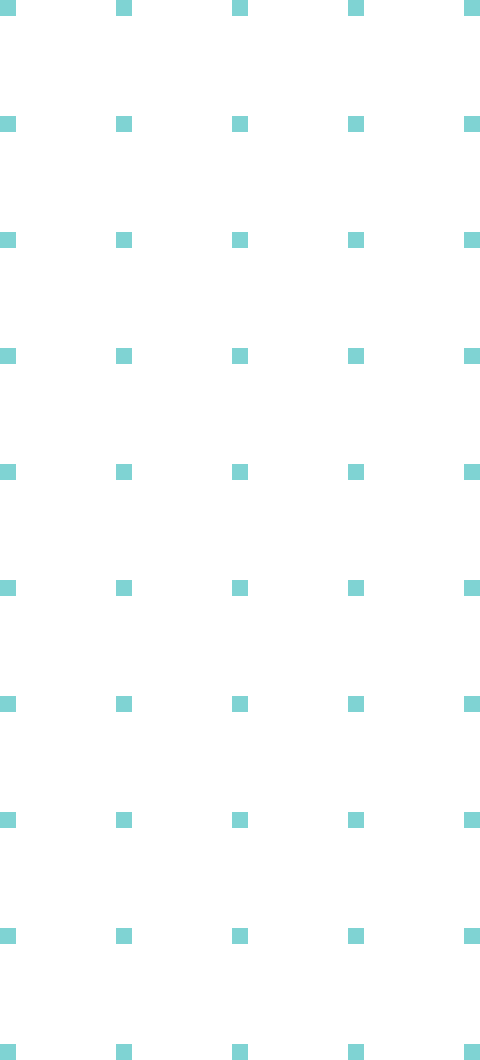
Media Bias
Curtailing bias and providing more effective search results.
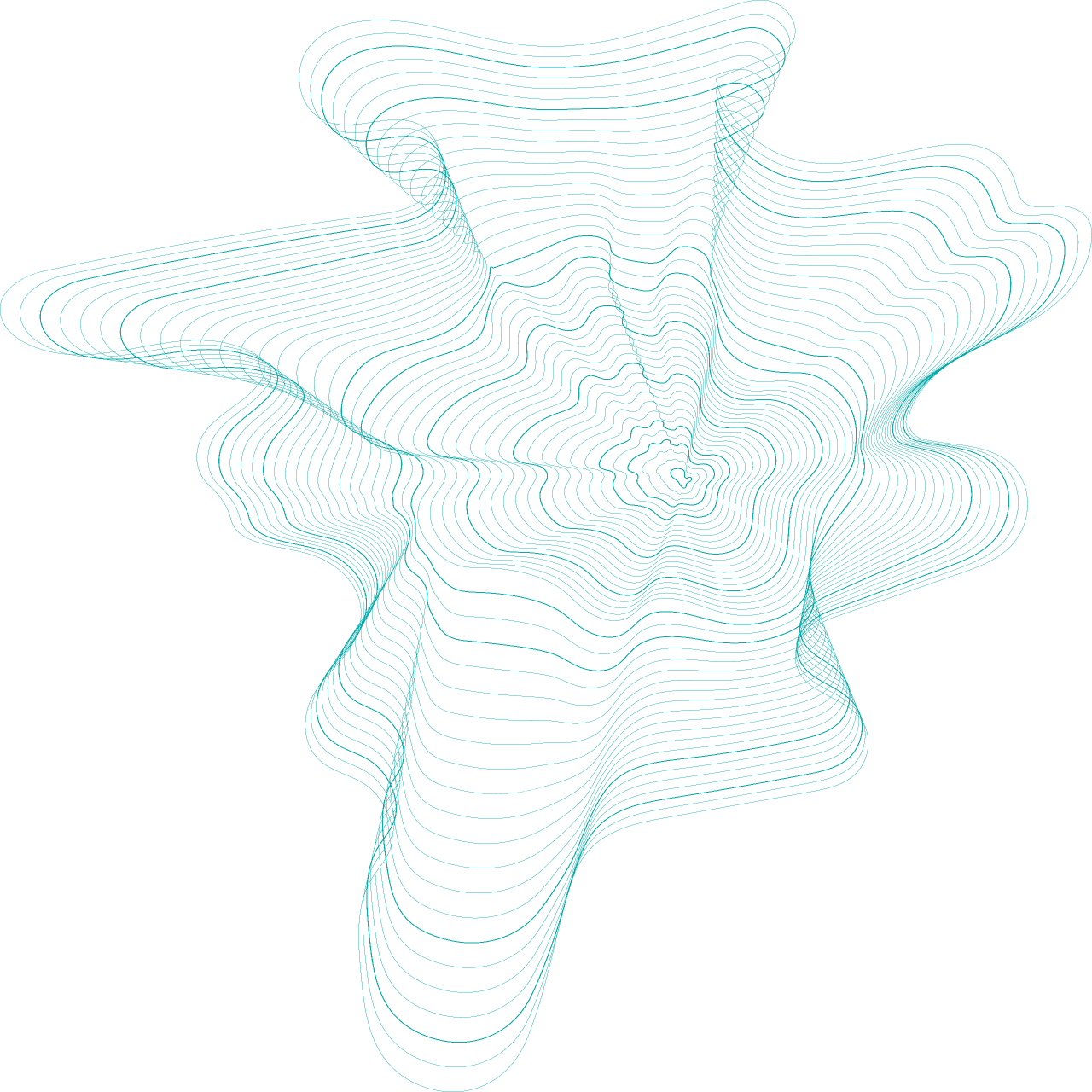
Online penetration and growth of online content is increasing at a rapid rate, 46% penetration, and 10% y-o-y growth as of 2016. However, as seen in recent news (e.g., Facebook’s interference with US elections), users are being subject to biased/nonneutral information.
Only 50% of U.S. adults feel confident there are enough sources to allow people to cut through bias in the news (down from 66% a generation ago). Therefore, it is critical to enable users and consumers of content to access neutral unbiased information so that they can make decisions based on true facts.
Every news story or article is stained by the bias of an author’s experiences, judgments, and predispositions. As humans, we communicate emotional statements as well as states of the world, and the way we choose to say things can often influence user experience. Since the 2016 presidential election, the number of searches for “Fake News” has increased ~25 fold.
The US media ranks worst in the world and surprisingly, half of Americans believe that online news websites report fake news regularly. For this reason, we wanted to identify and prevent the spread of misinformation online by quantifying bias, working closely with the AI for Good Foundation.
We proposed a model that predicts articles’ “bias score” based on selected features. The score encourages readers to be more critical and attempts to mitigate the negative effects of bias.
Scope
How might we leverage ML to reduce bias and more effectively present search results on specific topics?
Goals
Make the world a more trustworthy place through AI.
Methodology
Manual scoring
- Manual evaluation of over 240 news articles from different sources, from 0 to 5 (5 – unbiased/factual, 0 – completely biased).
Visualization strategy
- Determine features of interest to forecast the score of any article. Case study of a corpus of articles
Data Model Architecture
Features
- 3 manual scores
- Alexa’s country ranking of the source
- Subjectivity score of the title (NLTK)
- # of articles published by the source
- # of other sources mentioned in the body
Data Model Architecture
Visualization strategy
- Data cleaning – NumPy and Pandas
- Modeling
- Training set: 80%, Random forest: 500 trees, Cross-validation: 4-fold
Research Team
- Ana Luiza Ferrer
- Asef Ali
- David Huh
- Hugo Roucau
- Myriam Amour
- Shivam Mistry
Media Bias is part of an AI for Good project series, between the AI for Good Foundation and the Applied Data Science with Venture Applications Course at SCET, UC Berkeley.
Explore more SDG Launchpad Projects
Share this Page
Get Involved
Join our efforts to unlock AI’s potential towards serving humanity.
Support us
Become a Partner
Volunteer with us
Newsletter
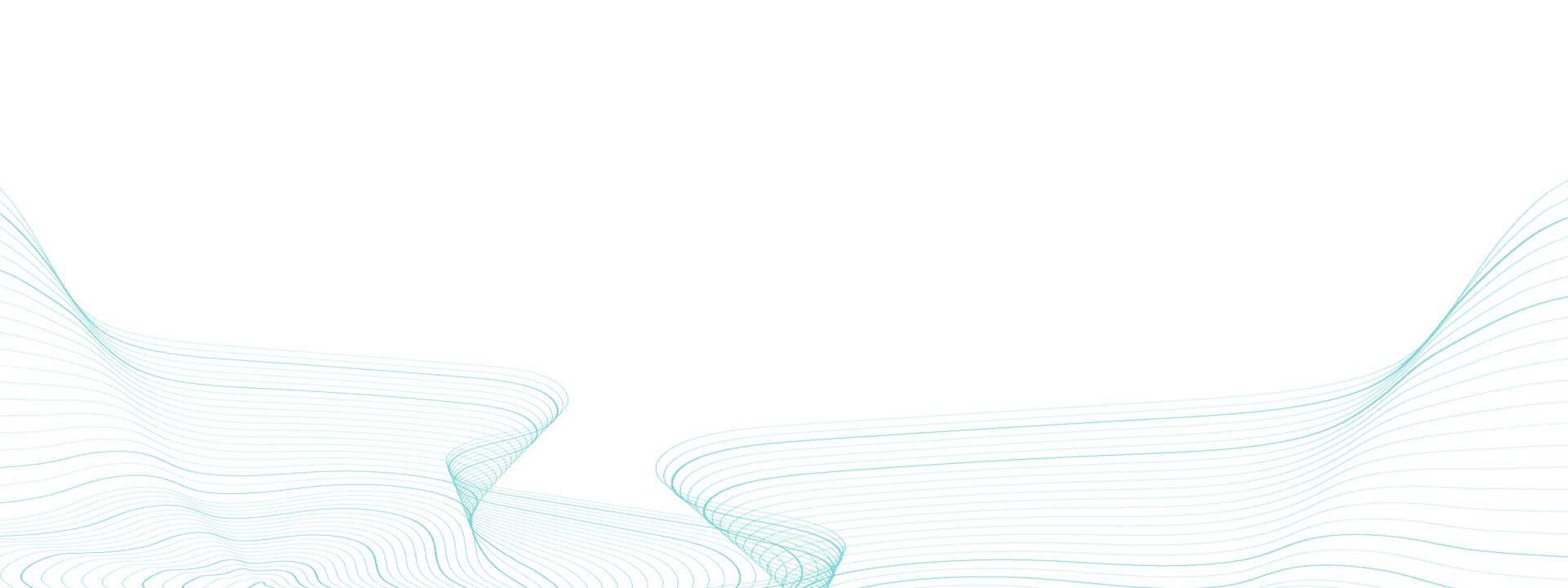